Yan Scholten
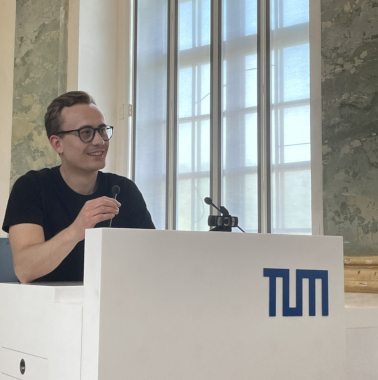
I am a PhD student in the Data Analytics and Machine Learning group at the Technical University of Munich (TUM) under the supervision of Prof. Stephan Günnemann. My research objective is to enhance the reliability of machine learning models. My broader research interests include adversarial robustness, uncertainty quantification, conformal prediction, machine unlearning, LLMs, and machine learning on graphs.
I started my PhD in 2022 after graduating from TUM in Informatics (M.Sc.) with high distinction, and from Paderborn University in Computer Science (B.Sc.) with a Minor in Mathematics (with distinction). Since 2023 I am associated to the Konrad Zuse School of Excellence in Reliable AI.
Selected Publications (full list)
- Hierarchical Randomized Smoothing
Real-world data is complex and often consists of objects that can be decomposed into multiple entities (e.g. images into pixels, graphs into interconnected nodes). Randomized smoothing is a powerful framework for making models provably robust against small changes to their inputs – by guaranteeing robustness of the majority vote when randomly adding noise before classification. Yet, certifying robustness on such complex data via randomized smoothing is challenging when adversaries do not arbitrarily perturb entire objects (e.g. images) but only a subset of their entities (e.g. pixels). As a solution, we introduce hierarchical randomized smoothing: We partially smooth objects by adding random noise only on a randomly selected subset of their entities. By adding noise in a more targeted manner than existing methods we obtain stronger robustness guarantees while maintaining high accuracy. We initialize hierarchical smoothing using different noising distributions, yielding novel robustness certificates for discrete and continuous domains. We experimentally demonstrate the importance of hierarchical smoothing in image and node classification, where it yields superior robustness-accuracy trade-offs. Overall, hierarchical smoothing is an important contribution towards models that are both – certifiably robust to perturbations and accurate. - Provable Adversarial Robustness for Group Equivariant Tasks: Graphs, Point Clouds, Molecules, and More
A machine learning model is traditionally considered robust if its prediction remains (almost) constant under input perturbations with small norm. However, real-world tasks like molecular property prediction or point cloud segmentation have inherent equivariances, such as rotation or permutation equivariance. In such tasks, even perturbations with large norm do not necessarily change an input’s semantic content. Furthermore, there are perturbations for which a model’s prediction explicitly needs to change. For the first time, we propose a sound notion of adversarial robustness that accounts for task equivariance. We then demonstrate that provable robustness can be achieved by (1) choosing a model that matches the task’s equivariances (2) certifying traditional adversarial robustness. Certification methods are, however, unavailable for many models, such as those with continuous equivariances. We close this gap by developing the framework of equivariance-preserving randomized smoothing, which enables architecture-agnostic certification. We additionally derive the first architecture-specific graph edit distance certificates, i.e. sound robustness guarantees for isomorphism equivariant tasks like node classification. Overall, a sound notion of robustness is an important prerequisite for future work at the intersection of robust and geometric machine learning. - Randomized Message-Interception Smoothing: Gray-box Certificates for Graph Neural Networks
Randomized smoothing is one of the most promising frameworks for certifying the adversarial robustness of machine learning models, including Graph Neural Networks (GNNs). Yet, existing randomized smoothing certificates for GNNs are overly pessimistic since they treat the model as a black box, ignoring the underlying architecture. To remedy this, we propose novel gray-box certificates that exploit the message-passing principle of GNNs: We randomly intercept messages and carefully analyze the probability that messages from adversarially controlled nodes reach their target nodes. Compared to existing certificates, we certify robustness to much stronger adversaries that control entire nodes in the graph and can arbitrarily manipulate node features. Our certificates provide stronger guarantees for attacks at larger distances, as messages from farther-away nodes are more likely to get intercepted. We demonstrate the effectiveness of our method on various models and datasets. Since our gray-box certificates consider the underlying graph structure, we can significantly improve certifiable robustness by applying graph sparsification.